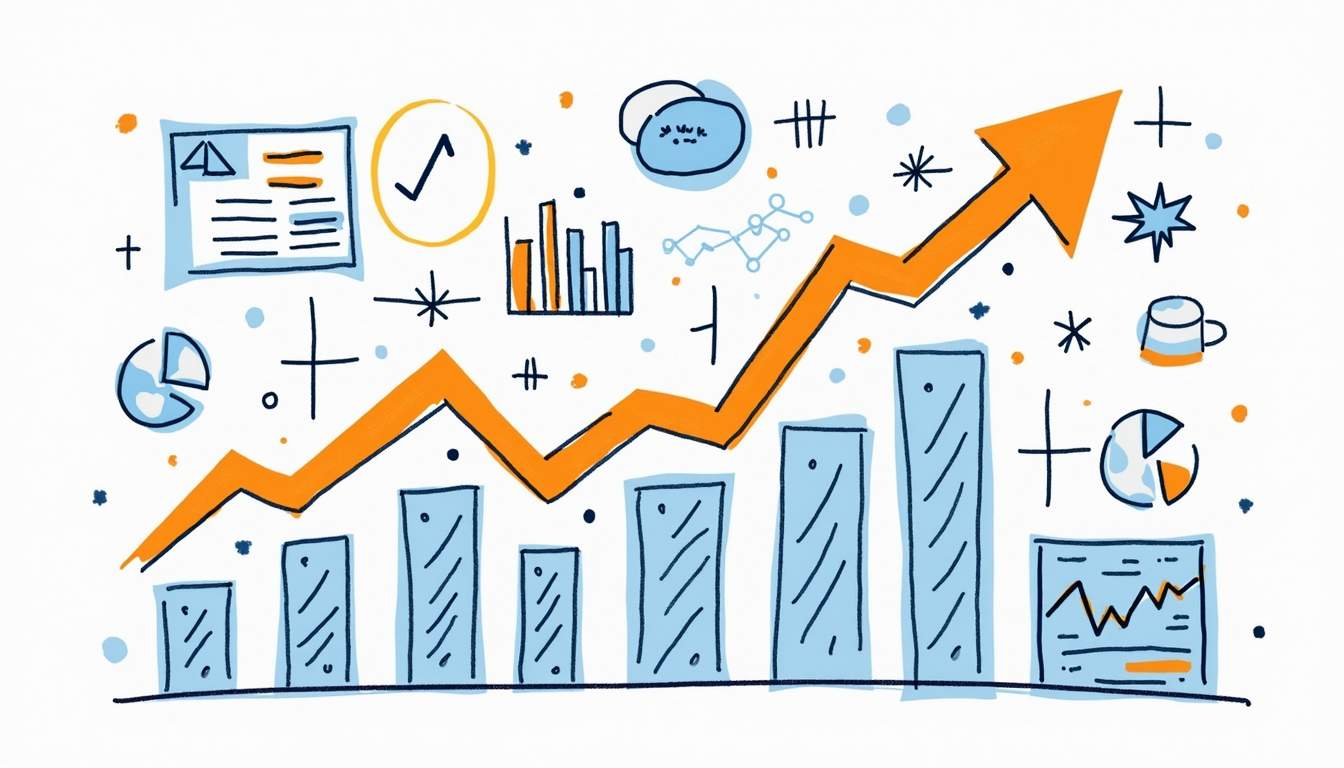
Data-Driven Lead Scoring Models: What You Need to Know
Understanding Lead Scoring
In the world of sales and marketing, lead scoring has become a vital strategy for prioritizing potential customers. Essentially, lead scoring involves assigning values to leads based on various attributes and behaviors, which helps businesses identify which prospects are most likely to convert. But what does it mean to be data-driven in this context?
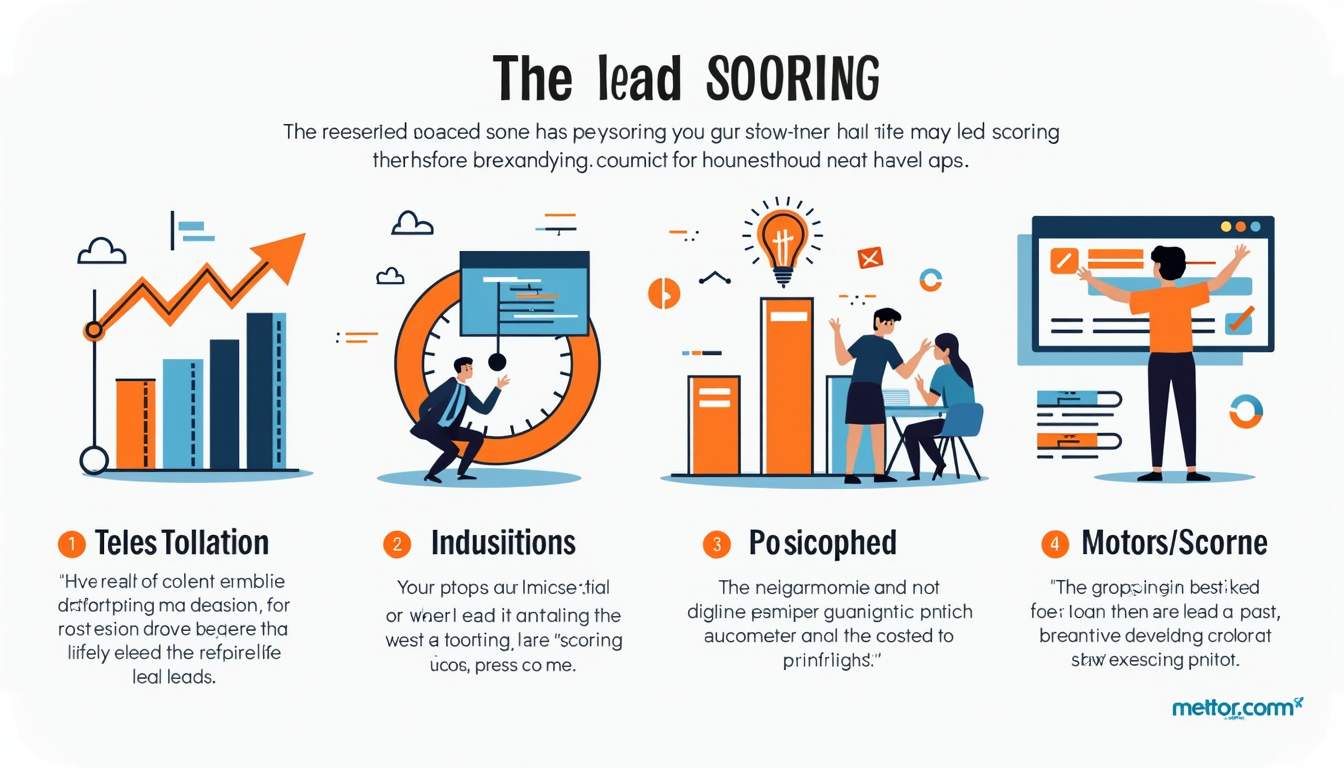
Data-driven lead scoring models leverage quantitative data to evaluate and rank leads. This means using actual metrics and analytics rather than relying solely on intuition or gut feelings. With the right approach, businesses can optimize their sales efforts and focus on leads that show genuine promise.
The Importance of Data in Lead Scoring
Data is the backbone of effective lead scoring. By harnessing data from multiple sources, companies can create a more accurate picture of their leads. This can include demographic information, engagement metrics, and even historical interactions with the brand.
For instance, a lead who frequently visits your website, engages with your content, and shares your posts on social media is likely more interested in your offerings than someone who has only filled out a contact form. By analyzing these behaviors, businesses can assign higher scores to more engaged leads, ensuring that sales teams focus their efforts where they matter most.
Types of Data to Consider
When building a data-driven lead scoring model, it's essential to consider various types of data. This can be broadly categorized into two groups: demographic and behavioral data.
Demographic data includes information such as age, location, job title, and company size. This data helps businesses understand who their ideal customers are. On the other hand, behavioral data provides insights into how leads interact with the brand, such as email open rates, website visits, and social media engagement. Combining these two types of data creates a more holistic view of each lead.
Moreover, it's important to recognize that lead scoring is not a static process. As market dynamics change and customer preferences evolve, the criteria used for scoring should also be revisited and adjusted accordingly. For instance, a lead that once showed minimal interest may become more engaged due to a new product launch or a targeted marketing campaign. Regularly updating your lead scoring model ensures that it remains relevant and effective, allowing your sales team to adapt their strategies in real-time.
Additionally, incorporating advanced analytics and machine learning can further enhance lead scoring efforts. By analyzing patterns and trends in large datasets, businesses can uncover hidden insights that traditional methods might overlook. This not only improves the accuracy of lead scoring but also enables predictive scoring, where businesses can anticipate future behaviors based on past interactions. Such sophisticated approaches can significantly boost conversion rates and lead to more successful sales outcomes.
Building a Data-Driven Lead Scoring Model
Creating a lead scoring model may seem daunting, but breaking it down into manageable steps can make the process smoother. Here’s a simple guide to get started.
Step 1: Define Your Ideal Customer Profile
The first step in building a lead scoring model is to define your ideal customer profile (ICP). This involves identifying the characteristics of your best customers. What industries do they belong to? What challenges do they face? What solutions do they seek? Understanding these factors will help you tailor your scoring criteria effectively.
Step 2: Choose Your Scoring Criteria
Once you have a clear ICP, it’s time to select the criteria for scoring leads. This can include both demographic and behavioral attributes. For example, you might assign points for specific job titles, industry relevance, or actions taken on your website.
It’s important to be strategic about your scoring system. Assign higher points to attributes that are more indicative of a lead’s likelihood to convert. This way, you can ensure that your sales team is focusing on leads that are genuinely interested in your products or services.
Step 3: Implement and Test Your Model
With your scoring criteria in place, the next step is to implement your model. This typically involves integrating your lead scoring system with your customer relationship management (CRM) software. Once set up, it’s crucial to monitor the performance of your model.
Testing is key. Analyze conversion rates and feedback from your sales team to determine if your scoring model is effective. If certain leads are consistently falling through the cracks, it may be time to revisit your scoring criteria and make adjustments.
Leveraging Technology for Lead Scoring
In today’s digital landscape, technology plays a significant role in lead scoring. Various tools and platforms can help automate the process, making it easier for businesses to manage and analyze their leads.
CRM Systems
Many CRM systems come equipped with built-in lead scoring features. These tools can automatically assign scores based on predefined criteria, allowing sales teams to focus on high-priority leads without manual intervention. This not only saves time but also ensures consistency in scoring.
Analytics Tools
Analytics tools can provide deeper insights into lead behavior. By tracking how leads interact with your content, these tools can help refine your scoring model over time. For example, if you notice that leads who download a specific resource are more likely to convert, you can adjust your scoring to reflect this behavior.
Machine Learning and AI
As technology continues to evolve, machine learning and artificial intelligence are becoming increasingly integrated into lead scoring models. These advanced technologies can analyze vast amounts of data to identify patterns and predict lead behavior more accurately. By leveraging AI, businesses can stay ahead of the curve and continuously improve their lead scoring processes.
Challenges of Data-Driven Lead Scoring
While data-driven lead scoring offers numerous benefits, it’s not without its challenges. Understanding these hurdles can help businesses navigate the complexities of implementing an effective scoring model.
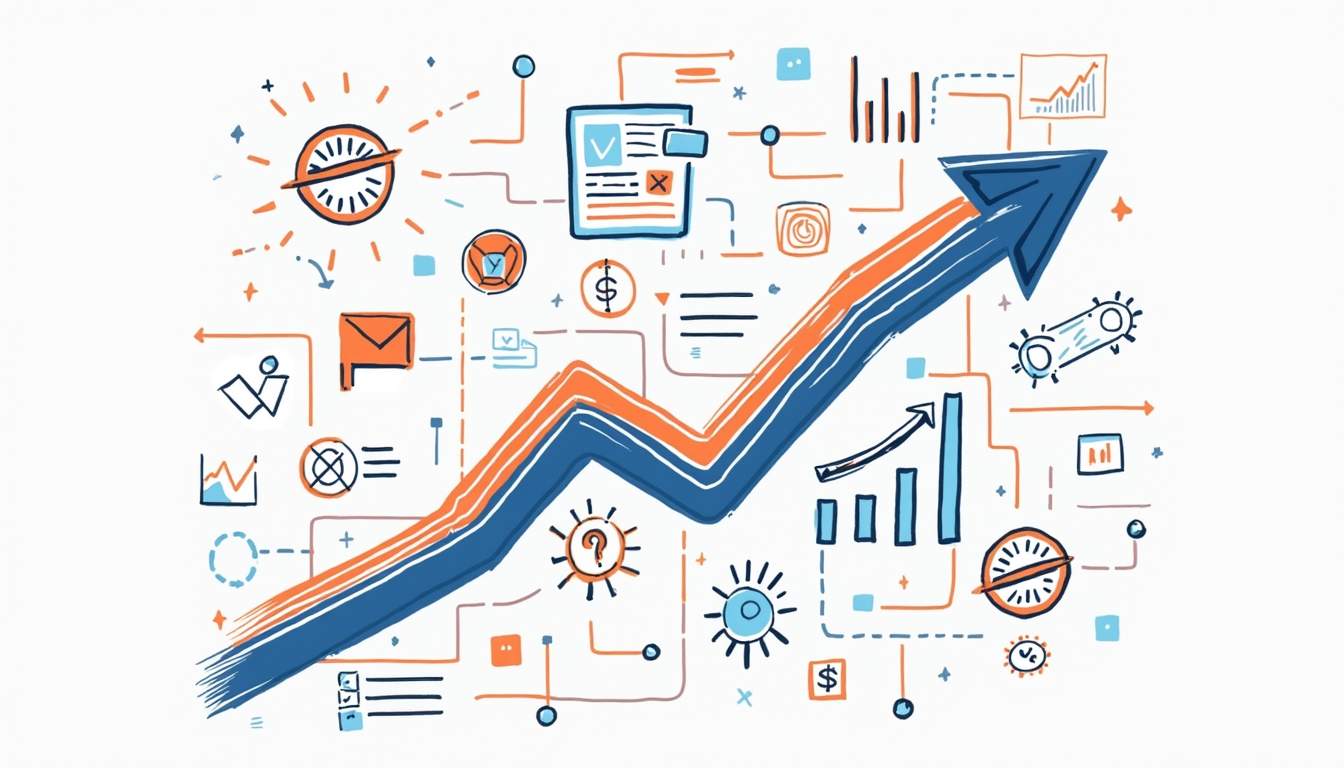
Data Quality
One of the most significant challenges is ensuring data quality. Inaccurate or outdated information can lead to misguided scoring and lost opportunities. Regularly cleaning and updating your data is essential to maintain the integrity of your lead scoring model.
Overcomplicating the Model
Another common pitfall is overcomplicating the scoring model. It can be tempting to include numerous criteria, but this often leads to confusion and inefficiency. A streamlined model with clear, actionable criteria is more effective than a convoluted one.
Resistance to Change
Finally, implementing a new lead scoring model may face resistance from team members accustomed to traditional methods. Providing training and demonstrating the benefits of a data-driven approach can help ease this transition and foster a culture of data-driven decision-making.
Conclusion
Data-driven lead scoring models are transforming the way businesses approach sales and marketing. By leveraging data to prioritize leads, companies can improve conversion rates and maximize their resources. While there are challenges to consider, the benefits of a well-implemented lead scoring system far outweigh the drawbacks.
In a competitive landscape, adopting a data-driven approach to lead scoring is not just an option; it’s a necessity. By understanding your leads better and focusing on the right prospects, businesses can drive growth and achieve their sales goals more effectively.
Ready to harness the power of data-driven lead scoring and transform your marketing strategy? At POP Advertising Partners, we specialize in utilizing high intent buyer data to streamline your customer acquisition and supercharge your paid ads and omnichannel marketing efforts. Let's Chat About Data & How It Can Help Your Business today, and take the first step towards optimizing your sales process and reducing your costs. Don't miss out on the opportunity to make data your most valuable sales partner.